What's ahead for big tech?
Shakeups can be expected, given that AI is in its early stages.
- A Chinese tech startup sent shockwaves through markets after revealing details of their free large-language model.
- After years of huge gains, US and other global tech companies most closely associated with AI saw a sharp pullback.
- Investors should not be surprised by quick shifts and new competitors in the AI space, given how early we are in our understanding of the technology.
Tech, power, and some other stocks were jolted on January 27 when a Chinese generative artificial intelligence (AI) company released the latest version of a free, large-language model (LLM). Many mega-cap tech stocks—some of the Magnificent 7 in particular—had their worst day in years. Since then, most of the hardest hit stocks recovered some of their losses.
Here’s what investors need to know about the latest AI news and how it might impact them.
Artificial intelligence competitor emerges
A foreign startup launched its V3 LLM back in December. On January 27, the same company released its new R1 model (which was built upon the V3 model) and quickly became a top downloaded app.
The company reported that the open-source LLM reportedly cost just $5.6 million and it appears to be functionally competitive to other AI tools.1 That being said, that figure is related to the last training run of the model, and we don’t have a full accounting of the entire spend picture. In addition, the cost doesn’t incorporate the costs associated with the open-source LLM models that were used to train R1.
Speed to market was another factor that took some investors off guard. Instead of taking months or years to pretrain the LLM, as has been the case with other models, R1 reportedly took just 2 months. Again, it is worth noting that the model was built using more reinforcement learning—building on top of existing models—making it faster to train and more efficient than one with no previous versions.
The news reinvigorated conversations about AI spend and within the AI community about whether algorithmic efficiency, represented in this case by the R1 LLM, may be a more efficient approach than brute-force computational power. The latter has been a driving factor behind massive spending by hyperscalers on big-data centers, chips, and power generation.
Investing implications
The R1 model raised questions about the estimated cost of building out AI going forward. Due to the compute costs for the R1 LLM appearing to be a fraction of that of other large foundational model builders, semiconductor and hardware stocks (which have far outperformed the broad market over the past several years) were rattled upon the news. Some power stocks, which have benefited from computational energy needs, were also impacted.
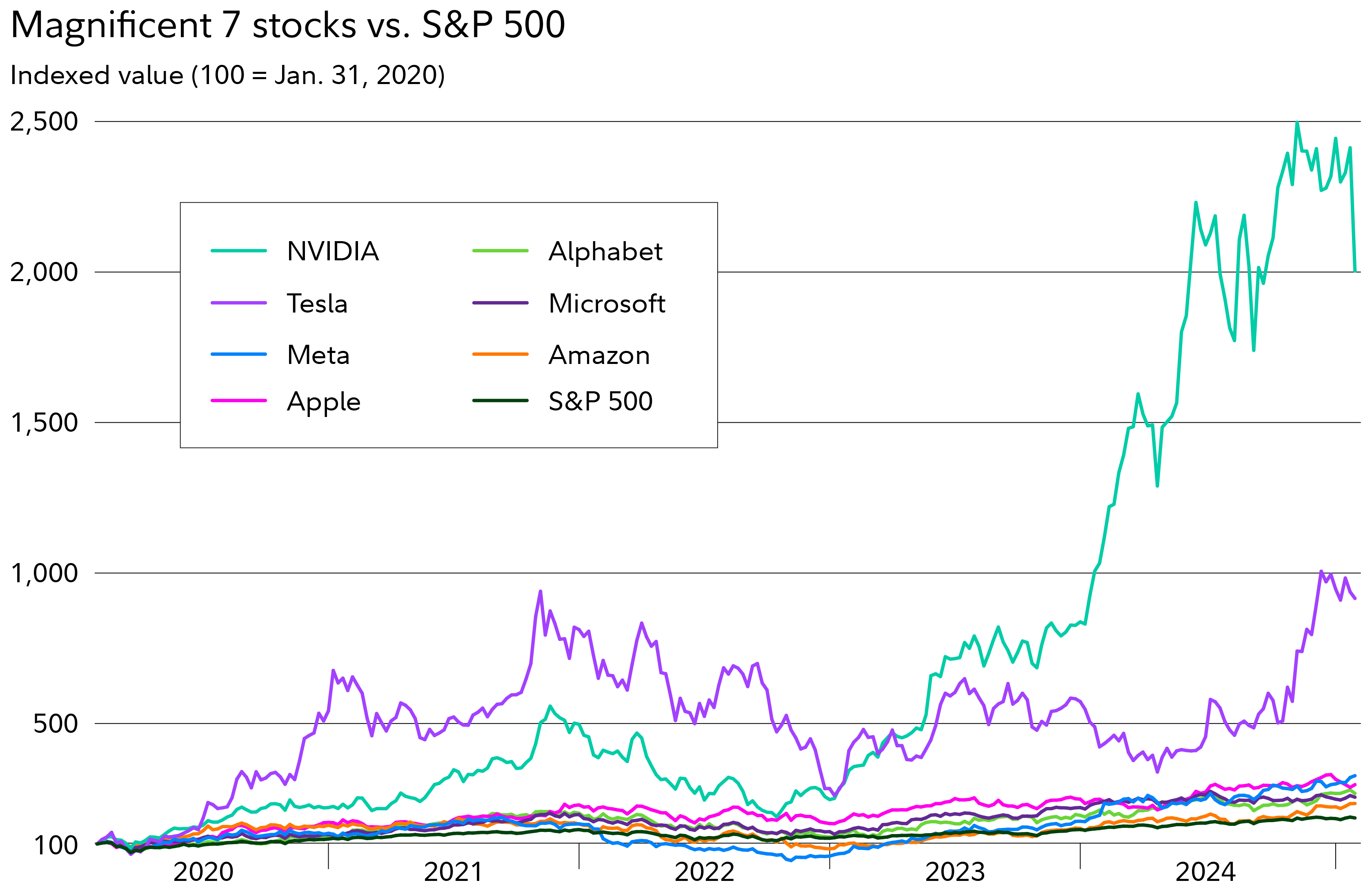
Source: FactSet, as of January 28, 2025.
While it’s still early, there was some rotation into software stocks from semiconductors, given the potential implication that new models could potentially be built on the backs of existing ones using more software and less hardware-intensive processes.
So, what does this mean for investors? Pri Bakshi, a portfolio manager at Fidelity, thinks this could have positive implications on AI adoption going forward, as investors may see more take-up in AI if it’s less expensive. However, he notes that we’re still very early in our understandings of AI, and we can expect the landscape for AI investing to continue to evolve over time.
Adam Benjamin, another portfolio manager at Fidelity, notes companies finding more efficient ways to train LLMs isn't a surprise, and investors should continue to expect companies to search for efficiencies. While some stocks sold off on concerns sold off on concerns that the models would require less compute, Benjamin thinks those companies can still offer value. R1 could accelerate the move from training to inference (i.e., using trained models to analyze new data and make predictions), which could drive a substantial increase in compute requirements as we are still in the early phases of the rollout of AI and its use cases.
Sector/Industry
Target specific segments of the economy with our full spectrum of sector funds, ETFs, and other solutions.
Learn more
Domestic Equity
Target your clients’ investment needs with our broad lineup of domestic equity solutions.
Learn more
Portfolio Construction Solutions
Get ready to manage your client portfolios—and relationships—more effectively with Fidelity's powerful portfolio construction insights, tools, and solutions.
Learn more
Before investing, consider the funds' investment objectives, risks, charges, and expenses. Contact Fidelity for a prospectus or, if available, a summary prospectus containing this information. Read it carefully.
1. Open source is software whose original source code is made freely available and may be redistributed and modified. And like other models that are more practical from a user perspective, R1 can also be post-trained (i.e., after the creator of the model pre-trains it, the user can train the model for a more specific task).
The S&P 500® Index is a market capitalization-weighted index of 500 common stocks chosen for market size, liquidity, and industry group representation to represent US equity performance.
Because of their narrow focus, sector investments tend to be more volatile than investments that diversify across many sectors and companies.
Stock markets are volatile and can fluctuate significantly in response to company, industry, political, regulatory, market, or economic developments. Investing in stock involves risks, including the loss of principal.
Foreign investments involve greater risks than U.S. investments, including political and economic risks and the risk of currency fluctuations, all of which may be magnified in emerging markets.
As with all your investments through Fidelity, you must make your own determination whether an investment in any particular security or securities is consistent with your investment objectives, risk tolerance, financial situation, and evaluation of the security. Fidelity is not recommending or endorsing this investment by making it available to its customers.
Past performance is no guarantee of future results.
Views expressed are as of the date indicated, based on the information available at that time, and may change based on market or other conditions. Unless otherwise noted, the opinions provided are those of the speaker or author and not necessarily those of Fidelity Investments or its affiliates. Fidelity does not assume any duty to update any of the information.
Fidelity Investments® provides investment products through Fidelity Distributors Company LLC; clearing, custody, or other brokerage services through National Financial Services LLC or Fidelity Brokerage Services LLC; and institutional advisory services through Fidelity Institutional Wealth Adviser LLC.