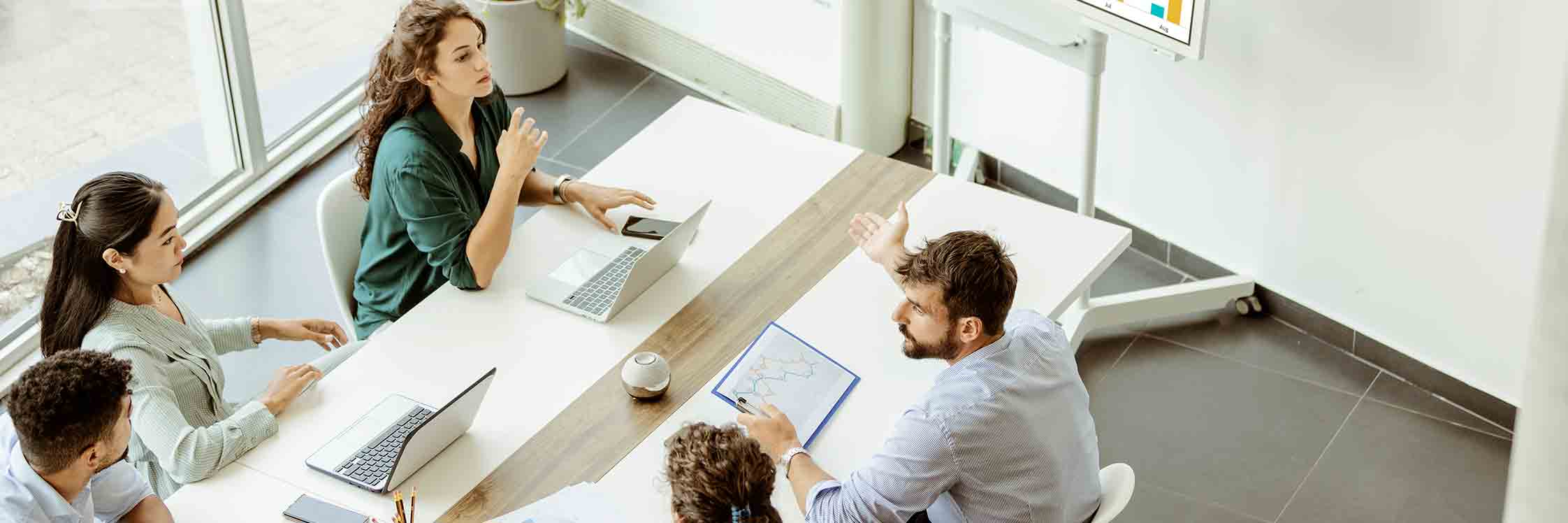
In a recent Market Insights Podcast, Denise Chisholm, director of quantitative market strategy in the Quantitative Research and Investments (QRI) division and Satya Chandrashekar, head of systematic equity research offer insight into the construction of a quantitative stock selection model.
Key Takeaways
- Systemic analysis of correlation, dispersion and statistical factors enhances portfolio construction.
- Our proprietary model includes more than 40 signals that are rigorously tested to optimize efficiencies and systematize the processes.
- Portfolio simulations using quant techniques enable return-optimizing risk management, keying in on when it pays to take larger bets and when to reduce risk in the face of headwinds.
Quantitative Stock Selection Model
Research is at the heart of our stock selection model process. Our research team generates multiple ideas through a robust, adaptable process, analyzing security-level risk through the lens of individual behavioral trends and geopolitical conditions. We employ these signals in a disciplined and systematic process, including portfolio simulation, analysis of dispersion, correlation, and economic factors.
Alpha Model Signals
We have developed approximately 40 signals in our proprietary quantitative stock selection model based on over 15 years of quantitative investing and 30 years of historical model data. These signals are categorized into various factor families, such as cyclical value, defensive value, growth, quality, momentum, and information flow. Each of these factor groups capture different dimensions of stock return forecasts. Our model isn't static. We continually search for new signals and factors to incorporate into our stock return forecasts. When we identify a potential return indicator, we rigorously test the efficacy of that signal. The signal we are testing must have sound economic rationale for why it forecast returns and how it adds value. We want the signal to be persistent through time and pervasive in different geographies. Only when the signal passes our threshold of econometric rigor will we add it into a model.
Portfolio Simulations and Patterns in Model Performance
Our portfolio simulations are run with real-life portfolio constraints, which is extremely important as that will inform us of the hypothetical performance had we been running a stock selection strategy since the onset of our data series, the early nineties. Successfully understanding when our strategy performs well in an investment cycle allows us to take larger bets in our portfolios when it pays to do so and take fewer bets when we face headwinds.
The average annualized excess returns using our model would be around 2.4% per year versus the benchmark. Over the last 10 years, the average annualized excess returns are slightly lower, but still quite strong at 1.7% a year. It is key to seek to understand under what regimes our model performs well and when our model might perform lower than our long-term return expectations. Comparing the performance of our model across different parts of the business cycle, we find that our model does much better in recessions. Long-run excess return expectations are about 2.4% a year; we see our models deliver annual excess returns of close to 3.6% during those periods. In a late cycle, we experience below average returns of about 1.2%. Again, this quantitative assessment informs our strategy.
Dispersion
Another strength of our model is its performance during periods of high cross-sectional return dispersion, that is, times where there's a big gap in the returns offered by the market's winners and losers. In a high-dispersion regime, the model correctly differentiates stocks among each other and gets rewarded for it. When dispersion of returns is tight, the model behaves differently. Take a hypothetical extreme case when the dispersion of returns is at or near zero. That means that whatever stock you select, you receive almost exactly the same returns. In such an environment, it is very hard to obtain outperformance through stock selection, and we'd expect our model performance would be below average. And this is exactly what we find in the data. In a high dispersion regime, our model earns double the returns compared to a low-dispersion regime.
Correlation
We see similar behavior from our model when it comes to average pairwise correlation. In a high correlation regime, all stocks are moving up and down together in lockstep. Our model has a harder time differentiating between stocks, and performs below average. But when the correlations between stocks is low, our model delivers above average returns—about 3% a year above its benchmark.
Statistical Factors
Another important aspect of model construction is the use of statistical factors to accurately forecast performance during periods of high market volatility, like an oil shock, pandemic, or a sudden shift in Fed policy. Analyzing underlying economic trends alone might not capture the factors driving returns during such periods. We find it's important to include a variety of statistical factors in our analysis to help model returns during such periods. And in this environment, our model tends to do better than average. Sometimes, when something truly unique occurs that's impacting the market, there may be only one or two statistical factors driving the majority of returns. In those cases, our model is slightly below average in long-term return forecasts.