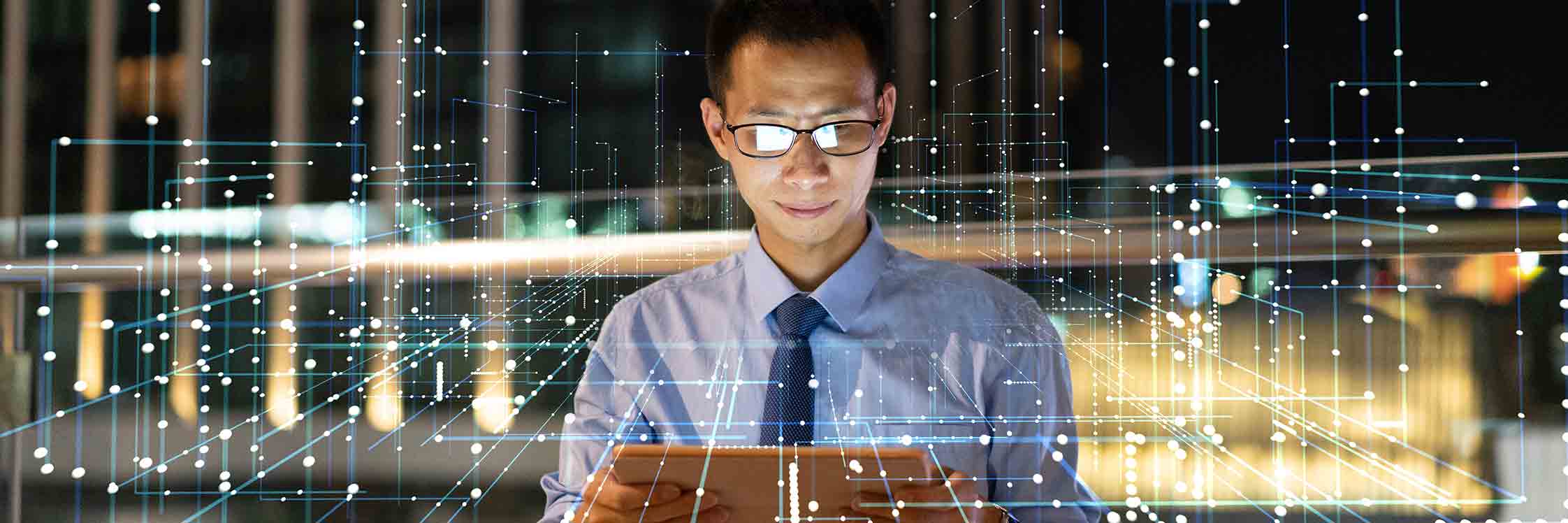
In recent Market Insights Podcasts, Denise Chisholm, director of quantitative market strategy in the Quantitative Research and Investments (QRI) division, Victor Liu, quantitative analyst, and Eric Khatchadourian, director of data analytics, discuss their framework on employing systematic research to offer data-driven insights into the portfolio construction process.
Key takeaways
- Systematic solutions are at the root of quantitative investing.
- Inputs, models and outputs contribute to predictive security selection.
- There are new possibilities to unlock hidden resources in data acquisition.
- Industry rotation models help to navigate volatility.
A Framework of Systematic Solutions
Fundamental analysis and quantitative analysis have long been regarded as two separate worlds. But approaches to data gathering and analysis have advanced very rapidly in recent years, and such quant techniques are proving fruitful when it comes to bolstering bottom-up fundamental analysis. In today's data-rich investing environment, fresh investment approaches require leveraging quantitatively disciplined solutions. Creating a framework of systemic solutions is at the root of quantitative investing.
Inputs, Models, and Outputs
How does a company make money? How will the issuer cover its interest payments? What do any of these variables look like in one year, five years? Systemic solutions are an engineered investment research approach which uses data inputs to model such questions and output an investment recommendation. Inputs may include the fundamentals of the company or issuer, the sector, the macro variables, or alternative data such as meeting notes with management, press releases, etc. The model reflects the newly captured understanding of the inputs. Well-engineered models display the capability to predictively identify stock that may have a higher than average probability of overperforming a benchmark.
New Possibilities with Data Acquisition
New approaches to data acquisition allow us to proxy or estimate hidden variables that cannot be observed directly. Engaging our platforms and projects to use the data, we construct our models, multi-factor alpha and proprietary risk models. We seek out the data that can adjust or reframe thinking, being careful researchers not to slip into confirmation bias from data mining. On the quantitative research front, there is a continual evaluation of new and interesting sources, while also looking at traditional data sources in new ways and methods. Advancements in natural language processing are allowing massive amounts of unstructured data to be captured and used in ways that just weren't possible before. Traditional trend-plotting or regression analysis can be joined with techniques such as decision trees or clustering algorithms in the modelling process, unlocking new possibilities.
Quantitative investment analysis is purely data driven and leveraged across strategies for our many active and enhanced index products and separately managed accounts. Our quant professionals work with investment professionals from research teams to portfolio managers to CIOs, and our teams' focus is specialized, such as stock selection models, emerging technology or in alternative data and machine learning methods. We start by forming investment hypotheses and then we connect the relevant data with financial ratios and alternative data science tools and statistics. Using the models, we build our portfolios to meet our investment objectives and our constraints.
Navigating Volatility with Macro Indicators
Macro indicators are very important building blocks for our models. One interesting example is our industry rotation model, a model that aims to predict a three month forward return for all industries. There are mainly two categories of predictors. One is the performance of industries in the past month, i.e. cross industry momentum. The second category is the macro indicators. We look at different types of macro indicators including forecasts of GDP growth, inflation rate, unemployment rate, and many others. From the two categories of predictors, we use historical data and statistical models to choose most relevant predictors and predict performance for each industry. An interesting finding is that historically, cross industry momentum is often the main driver for the prediction.
More recently, macro indicators have become more and more important. We retrain our model monthly in order to swiftly adapt to the challenging environment, revamping our pessimistic or optimistic predictive conclusions for each industry. For example, high inflation, changes in oil prices, and forecasted wage growth have broad cross-market impacts, but those impacts differ by industry, with some performing worse than others. Similarly, we can predict a better performance in other industries due to the reversal impact of the indicators.
Analysis Backed by Expertise
These datasets don't stand alone, and we are always striving to construct a complete and current understanding of the investment landscape. It is crucial to combine the data and analytics with subject matter expertise in the domain, to understand whether the mathematical functions are modelling the real underlying dynamics. Utilizing data points to validate our predictions and the expertise of our seasoned investment experts to drive a disciplined and ever-improving process, we gain and leverage useful data-driven insights into the investment process.